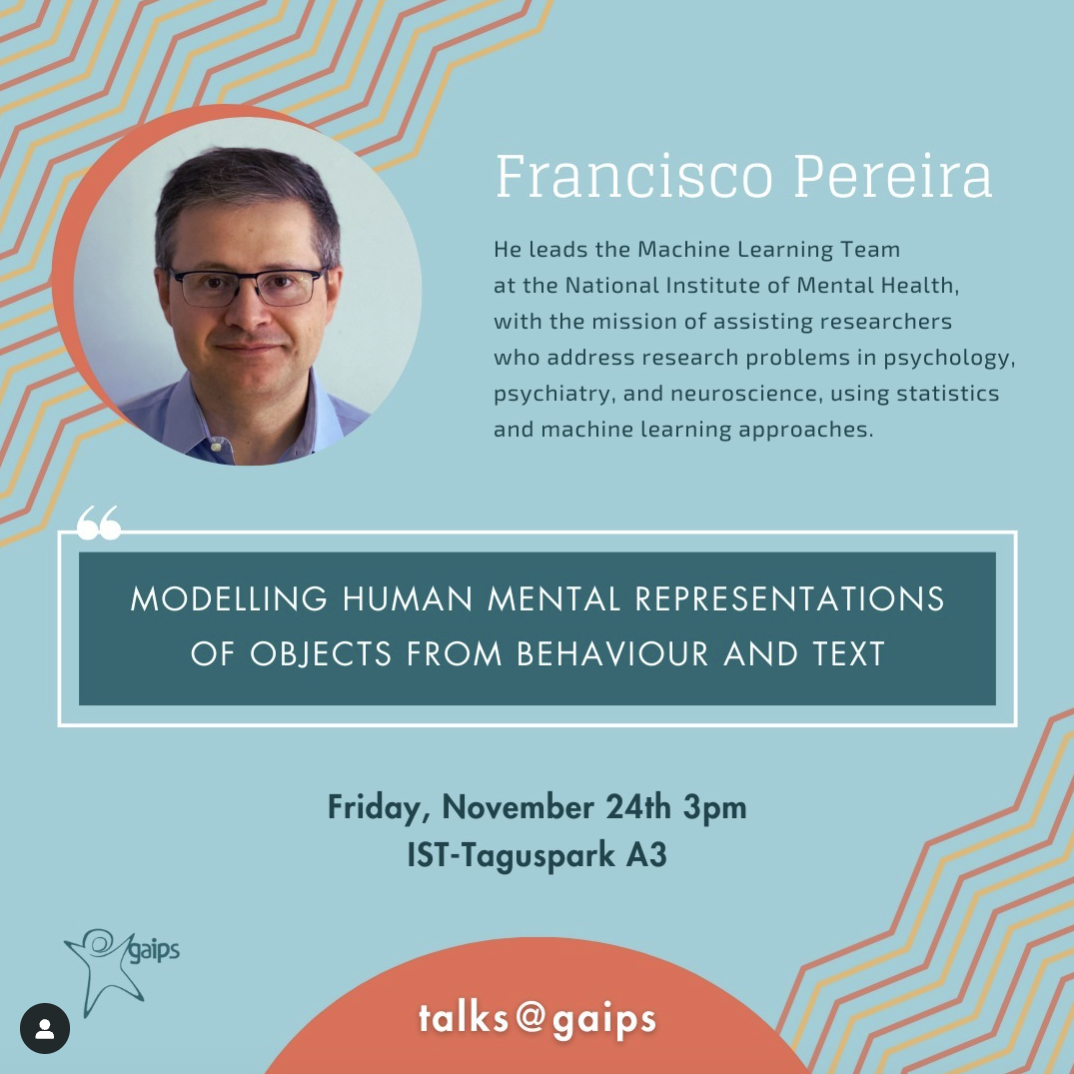
GAIPS Talk by Francisco Pereira (National Institute of Mental Health)
On November 24th at 3pm, INESC ID GAIPS Lab (Group of AI for People and Society) will host the talk “Modelling Human Mental Representations of Objects from Behavior and Text” by Francisco Pereira (National Institute of Mental Health).
Francisco Pereira is the leader of the Machine Learning Team at the National Institute of Mental Health and in this talk he will delve into the THINGS Initiative, an ongoing project collecting a vast behavioural dataset on object similarity judgments. Discover a novel machine learning approach unveiling the “core dimensions” of object representation and their impact on human interactions
The event will take place at Técnico – Taguspark (Room A3) and online via zoom.
Abstract
Modelling human mental representations of objects from behaviour and text:
Psychological research about mental representations of objects describes them at different levels of granularity, from coarse (e.g. animacy, shape, colour, function, taxonomical category) to fine (e.g. thousands of properties in norming studies). However, this does not necessarily tell us which of those characteristics are most important in our interactions with objects, across tasks and purposes. In the first part of this talk, I will describe the THINGS Initiative, an ongoing effort by our collaborators to collect a behavioral dataset of millions of odd-one-out similarity judgements on thousands of objects, in tandem with many complementary datasets (fMRI, MEG, EEG, norming studies). In the context of this project, we developed a novel machine learning approach to identifying the “core dimensions” of object representation used in those judgements. Our approach models each object as a sparse, non-negative embedding, and judgements as a function of the similarity of those embeddings. The resulting model predicts subject behaviour on test data, as well as the fine-grained structure of object similarity. The dimensions of the embedding space are coherently interpretable by test subjects, and reflect degrees of taxonomic membership, functionality, and perceptual or structural attributes, among other characteristics. Furthermore, naive subjects can accurately rate new objects along these dimensions, without training. In the second part of this talk, I will describe a parallel research effort to develop models of an object interaction space. In this project, we developed an approach to represent objects in a space where each dimension corresponds to a broad mode of interaction, based on action verb selectional preferences collected in text corpora. This embedding makes it possible to predict human judgments of verb applicability in new objects. The dimensions in this space can also be used to predict categorical and functional dimensions from the object representation derived from behaviour. Together, these results suggest that interaction knowledge accounts for much of the mental representations of objects.
Bio
Francisco Pereira leads the Machine Learning Team at the National Institute of Mental Health (https://cmn.nimh.nih.gov/mlt)
Papers about his work:
“Revealing the multidimensional mental representations of natural objects underlying human similarity judgements”
“VICE: Variational Interpretable Concept Embeddings”
“Mental representations of objects reflect the ways in which we interact with them”